How Machine Learning is Enhancing Cloud Platform Reliability and Uptime
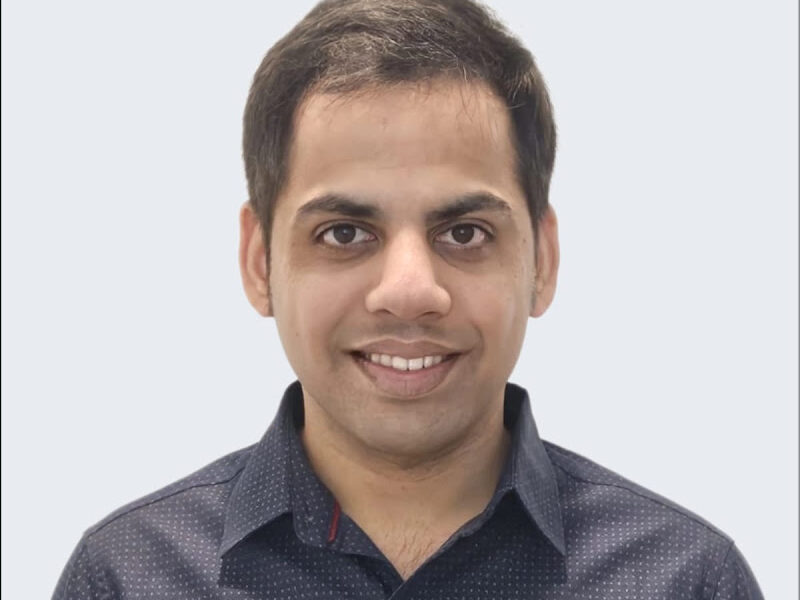
Cloud platforms have become the foundation of modern digital infrastructure, supporting businesses, applications, and services at an unprecedented scale. However, ensuring high reliability and uptime remains a major challenge due to the dynamic nature of cloud environments, increasing workloads, and the sheer complexity of distributed systems. Traditional monitoring and management techniques often fall short in predicting failures or optimizing resources in real time. To address these challenges, cloud providers and enterprises are turning to machine learning to enhance reliability, automate failure detection, and optimize system performance.
This article explores how machine learning is transforming cloud reliability by minimizing downtime, improving fault tolerance, and enabling predictive analytics for cloud operations.
The Growing Importance of Cloud Reliability
Cloud platforms are more complex than ever, with microservices architectures, containerized applications, and multi-region deployments generating vast amounts of telemetry data. As a result, manually monitoring cloud performance and anticipating failures has become nearly impossible. The cost of downtime continues to rise, with IT outages potentially leading to millions of dollars in financial losses, customer dissatisfaction, and operational disruptions. Traditional cloud management approaches rely on reactive measures, where failures are addressed only after they occur. Machine learning introduces a proactive approach, identifying potential risks before they escalate into major incidents.
Machine Learning for Predictive Maintenance and Failure Detection
One of the most impactful applications of machine learning in cloud reliability is predictive maintenance. By analyzing historical performance data, machine learning models can identify patterns that indicate an impending system failure. These models process telemetry data such as CPU usage, network latency, and disk performance to recognize deviations from normal behavior. Once an anomaly is detected, automated remediation mechanisms can be triggered, such as scaling up resources, reallocating workloads, or restarting malfunctioning services before users experience disruptions. Predictive maintenance reduces unplanned downtime and extends the lifespan of cloud infrastructure by preventing failures before they happen.
Anomaly Detection for Proactive Cloud Monitoring
Machine Learning plays a crucial role in anomaly detection by continuously monitoring cloud infrastructure for unusual behavior. Unlike traditional monitoring systems that rely on predefined thresholds, machine learning models adapt dynamically and detect subtle deviations that could indicate system degradation, security breaches, or misconfigurations. By leveraging historical performance data, these models can distinguish between expected fluctuations and genuine anomalies that require intervention. This capability enhances system stability, as anomalies can be addressed before they lead to service degradation or outages.
Automated Incident Response and Self-Healing Mechanisms
Machine learning is transforming incident response by reducing the time required to detect and resolve cloud failures. Instead of relying on human intervention, AI-driven incident management systems can analyze logs, identify root causes, and initiate corrective actions automatically. Self-healing cloud infrastructure leverages machine learning models to detect performance degradation, isolate affected components, and restore services with minimal disruption. Automated remediation strategies can include rolling back to previous stable configurations, reallocating workloads, or deploying security patches dynamically. These capabilities significantly improve mean time to resolution (MTTR) and ensure continuous service availability.
Optimizing Cloud Resource Allocation with AI
Cloud reliability is closely linked to efficient resource management. Over-provisioning cloud resources leads to unnecessary costs, while under-provisioning results in performance bottlenecks. Machine learning optimizes resource allocation by analyzing demand patterns and predicting workload fluctuations. AI-driven auto-scaling dynamically adjusts compute, storage, and network resources to meet application demands while minimizing overhead costs. By continuously learning from past usage trends, machine learning models ensure that cloud environments remain resilient, responsive, and cost-efficient.
Log Analysis and Root Cause Identification
Log analysis is a critical component of cloud reliability, as logs contain valuable insights into system performance, security incidents, and operational trends. However, analyzing vast amounts of log data manually is inefficient and time-consuming. Machine learning automates log analysis by identifying patterns and correlations across distributed cloud environments. Natural language processing (NLP) techniques extract key insights from logs, enabling faster root cause identification. When failures occur, AI-powered log analysis can pinpoint the underlying issue within seconds, allowing teams to resolve problems swiftly and prevent similar incidents in the future.
Challenges in Implementing Machine Learning for Cloud Reliability
Despite its advantages, implementing machine learning in cloud reliability comes with challenges. High-quality data is essential for training accurate models, but cloud environments generate noisy and inconsistent telemetry data, making data preprocessing a critical step. Machine learning models must also be fine-tuned to minimize false positives, as excessive alerts can lead to alert fatigue among engineers. Additionally, security risks such as adversarial attacks on AI models must be addressed to prevent malicious actors from manipulating system behavior. Overcoming these challenges requires continuous model refinement, integration with existing cloud security frameworks, and adopting explainable AI techniques to enhance trust in machine learning-driven decisions.
The Future of AI-Driven Cloud Reliability
The adoption of AI-powered reliability solutions in cloud computing is expected to accelerate in the coming years. AI-augmented cloud operations will enable greater automation, allowing cloud platforms to self-optimize and self-repair with minimal human intervention. Federated learning techniques will enhance collaborative cloud monitoring while maintaining data privacy across multi-cloud environments. The integration of large language models into cloud reliability workflows will further improve automated troubleshooting and incident resolution. As AI technology advances, cloud providers will continue leveraging machine learning to build more resilient, efficient, and secure cloud infrastructures.
Conclusion
Machine learning is revolutionizing cloud platform reliability by enabling predictive maintenance, intelligent anomaly detection, automated incident response, and optimized resource management. These advancements significantly reduce downtime, improve fault tolerance, and enhance overall cloud performance. As enterprises continue to rely on cloud infrastructure for mission-critical workloads, AI-driven reliability solutions will become essential for ensuring seamless operations. By embracing machine learning, organizations can create self-healing cloud platforms that proactively prevent failures, optimize performance, and deliver uninterrupted digital services.